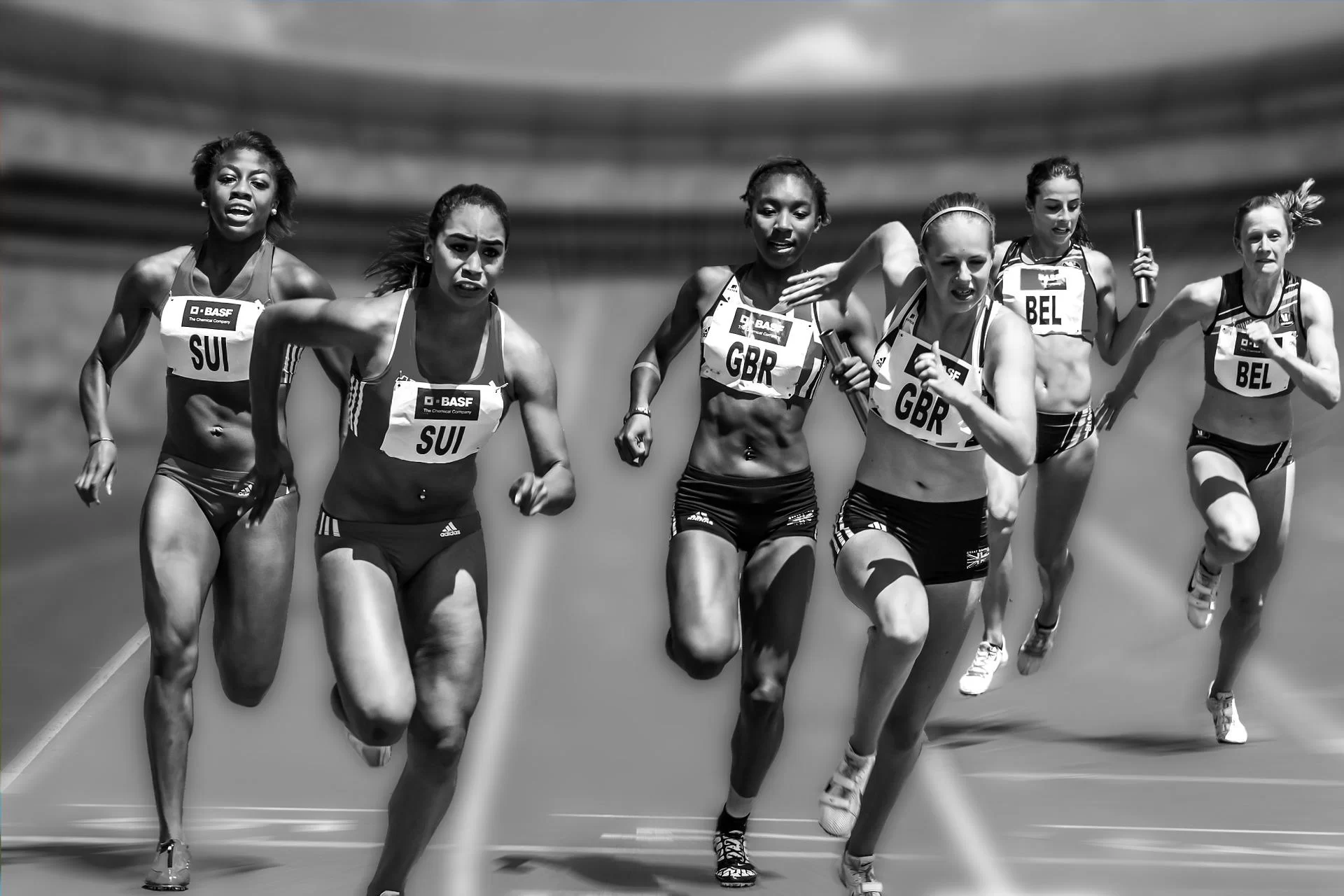
When is your AI system done?
Ensuring your system works for all edge cases can take considerable time and investment.
Ensuring your system works for all edge cases can take considerable time and investment.
There’s much similarity between testing applied AI/ML (For brevity, I’ll just refer to these as “AI”) applications and traditional software[1]. Regardless of the vertical and
Last week, I searched online for IT outsourcing advice to see what I would find. It was an eye-opening experience to see the topic is dominated by one thing – hourly rates.
The availability of pre-trained AI models is enabling lower cost, low risk development of AI applications.
AI systems are smart, but they do not learn after they are deployed. The “learning” part of an AI system happens during training. Once trained, the model is static until you retrain. Retraining can be expensive, time-consuming, and difficult because of the need for new, labeled data.
Many Artificial Intelligence initiatives are doomed from the start – but needn’t be. Here are five things that keep AI projects from achieving success. 1.
While hiring smart people and providing a sandbox environment seems an obvious place to begin, real-world AI initiatives gain little from sandbox innovation. An effective